
The Cutting Edge of Intelligenza Artificiale: Latest Developments and Future Trends
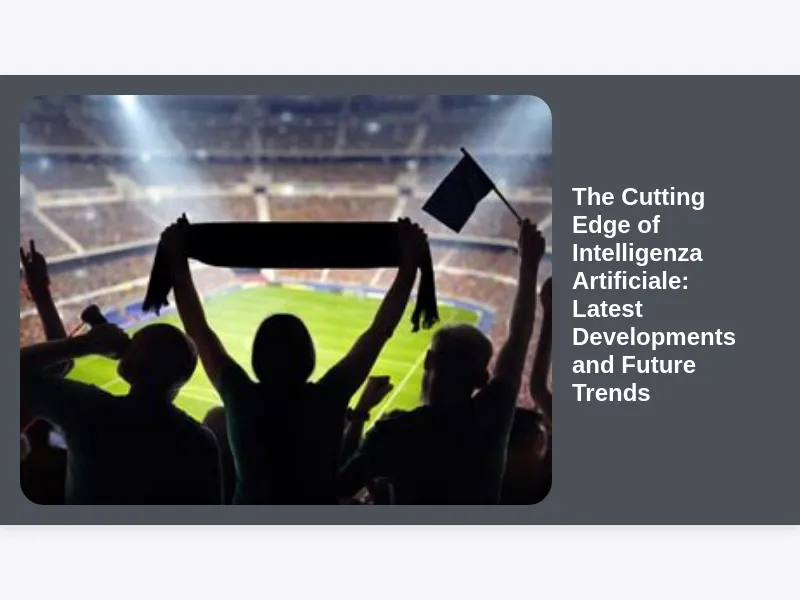
Intelligenza Artificiale (Artificial Intelligence), or AI, is no longer a futuristic concept relegated to science fiction. It's a rapidly evolving field permeating almost every facet of modern life, from the algorithms that curate our social media feeds to the sophisticated systems driving self-driving cars. But what exactly is the latest in Intelligenza Artificiale? This article delves into the cutting edge of AI, exploring recent breakthroughs, current applications, and future trends that are reshaping our world.
1. Generative AI: The Rise of Creative Intelligenza Artificiale
Generative AI is arguably one of the most impactful recent developments in Intelligenza Artificiale. These models, like DALL-E 2, Midjourney, and Stable Diffusion for image generation, and ChatGPT for text, have captivated the public with their ability to create entirely new content based on user prompts.
- How Generative AI Works: Generative AI models are typically based on deep learning architectures, particularly transformers. They are trained on massive datasets of images, text, or other data types, learning the underlying patterns and structures of the data. Once trained, they can generate new, original content that resembles the data they were trained on. For example, a text-based generative AI might generate human-quality text from a simple prompt.
- Applications: The applications of generative AI are vast and growing. In creative fields, it's being used for:
- Art generation: Creating original artwork, illustrations, and designs.
- Content creation: Writing articles, blog posts, marketing copy, and scripts.
- Music composition: Generating original melodies, harmonies, and arrangements.
- Video production: Creating animated videos, special effects, and even full-fledged movies.
- Beyond creative tasks, Generative AI is also being applied in:
- Drug discovery: Generating novel molecules with desired properties.
- Materials science: Designing new materials with specific functionalities.
- Software development: Generating code snippets and even entire applications.
2. Advancements in Natural Language Processing (NLP): Enabling Meaningful Interactions with Intelligenza Artificiale
Natural Language Processing (NLP) is a branch of Intelligenza Artificiale focused on enabling computers to understand, interpret, and generate human language. Recent advancements in NLP have led to significant improvements in machine translation, chatbots, and other language-based applications.
- Transformer Networks: The rise of transformer networks, such as BERT, RoBERTa, and GPT-3, has revolutionized NLP. These models excel at capturing long-range dependencies in text, allowing them to understand context and nuance more effectively.
- Contextual Understanding: Newer NLP models demonstrate a heightened ability to understand context. This means they can differentiate between multiple meanings of a word, interpret sarcasm, and even infer intent from user input.
- Applications:
- Improved Chatbots: NLP powers increasingly sophisticated chatbots capable of handling complex conversations and providing personalized customer service.
- Enhanced Machine Translation: Machine translation tools are becoming more accurate and fluent, breaking down language barriers and facilitating global communication.
- Sentiment Analysis: NLP is used to analyze text and identify the sentiment expressed (e.g., positive, negative, neutral), which is valuable for market research and brand monitoring.
- Text Summarization: NLP algorithms can automatically summarize long articles and documents, saving users time and effort.
3. Computer Vision: Intelligenza Artificiale That Can See and Understand
Computer vision is another crucial area of Intelligenza Artificiale, enabling computers to "see" and interpret images and videos. Recent advances have led to more accurate object recognition, image segmentation, and video analysis.
- Convolutional Neural Networks (CNNs): CNNs remain the dominant architecture for computer vision tasks. They are particularly well-suited for identifying patterns and features in images.
- Object Detection and Recognition: Computer vision systems can now accurately identify and classify objects in images and videos, even in complex scenes.
- Image Segmentation: Image segmentation involves dividing an image into different regions or segments, allowing for more precise analysis and understanding.
- Applications:
- Self-Driving Cars: Computer vision is essential for self-driving cars, enabling them to perceive their surroundings and navigate safely.
- Medical Imaging: Computer vision is used to analyze medical images, such as X-rays and MRIs, to detect diseases and anomalies.
- Facial Recognition: Facial recognition technology is used for security, access control, and other applications.
- Manufacturing: Computer vision is used for quality control in manufacturing, detecting defects and ensuring product quality.
- Retail: Analyzing customer behavior, improving inventory management, and preventing theft.
4. Reinforcement Learning: Training Intelligenza Artificiale Through Trial and Error
Reinforcement learning (RL) is a type of machine learning where an agent learns to make decisions in an environment by receiving rewards or penalties for its actions. It's particularly well-suited for training AI to perform complex tasks, such as playing games and controlling robots.
- How Reinforcement Learning Works: An RL agent interacts with its environment, taking actions and observing the results. Based on the rewards or penalties it receives, the agent learns to optimize its behavior to achieve a specific goal.
- Deep Reinforcement Learning: Combining reinforcement learning with deep learning has led to significant breakthroughs. Deep RL algorithms can handle more complex environments and learn more sophisticated strategies.
- Applications:
- Game Playing: RL has achieved superhuman performance in games like Go, chess, and video games.
- Robotics: RL is used to train robots to perform tasks such as grasping objects, walking, and navigating complex environments.
- Resource Management: RL is used to optimize resource allocation in areas such as energy management and traffic control.
- Finance: RL is used for algorithmic trading and portfolio management.
5. Edge AI: Bringing Intelligenza Artificiale to the Edge of the Network
Edge AI involves running Intelligenza Artificiale algorithms on devices at the edge of the network, rather than relying on centralized cloud servers. This offers several advantages, including reduced latency, increased privacy, and improved reliability.
- Benefits of Edge AI:
- Reduced Latency: Processing data locally eliminates the need to transmit data to the cloud, reducing latency and enabling real-time decision-making.
- Increased Privacy: Keeping data on-device protects sensitive information from being exposed to the cloud.
- Improved Reliability: Edge AI can operate even when there is no internet connection, ensuring continuous operation.
- Applications:
- Autonomous Vehicles: Edge AI is critical for autonomous vehicles, enabling them to process sensor data and make decisions in real time.
- Industrial Automation: Edge AI is used to monitor equipment, detect anomalies, and optimize processes in industrial settings.
- Smart Homes: Edge AI powers smart home devices that can understand and respond to user commands without relying on the cloud.
- Healthcare: Edge AI is used in medical devices to monitor patients' health and provide real-time alerts.
6. Explainable AI (XAI): Making Intelligenza Artificiale More Transparent and Understandable
As Intelligenza Artificiale becomes more prevalent, it's increasingly important to understand how AI models make decisions. Explainable AI (XAI) aims to develop AI systems that can explain their reasoning and decision-making processes in a way that humans can understand.
- Importance of XAI:
- Trust: Understanding how AI models work builds trust and confidence in their decisions.
- Accountability: XAI helps to identify and address biases in AI models, ensuring fairness and accountability.
- Regulation: XAI is essential for meeting regulatory requirements that mandate transparency in AI systems.
- Techniques for XAI:
- Feature Importance: Identifying which features have the most influence on an AI model's predictions.
- Rule-Based Explanations: Generating rules that describe how an AI model makes decisions.
- Visual Explanations: Using visualizations to explain the reasoning behind an AI model's predictions.
- Applications:
- Healthcare: Explaining how AI models diagnose diseases and recommend treatments.
- Finance: Explaining how AI models make credit decisions and detect fraud.
- Criminal Justice: Explaining how AI models are used in risk assessment and sentencing.
7. Quantum Computing and Intelligenza Artificiale: A Future Synergy
While still in its early stages, quantum computing holds immense potential to revolutionize Intelligenza Artificiale. Quantum computers can perform certain calculations much faster than classical computers, which could lead to breakthroughs in machine learning and optimization.
- Potential Benefits:
- Faster Training: Quantum algorithms could significantly speed up the training of AI models.
- Improved Optimization: Quantum computing could be used to find optimal solutions to complex optimization problems in AI.
- New AI Algorithms: Quantum computers could enable the development of entirely new AI algorithms that are not possible on classical computers.
- Current Research: Researchers are exploring the use of quantum computing for various AI tasks, including:
- Quantum Machine Learning: Developing quantum algorithms for machine learning tasks.
- Quantum Optimization: Using quantum computing to optimize the parameters of AI models.
- Quantum Neural Networks: Creating neural networks that run on quantum computers.
8. The Ethical Considerations of Intelligenza Artificiale: Ensuring Responsible Development and Deployment
As Intelligenza Artificiale becomes more powerful, it's crucial to address the ethical implications of its development and deployment. This includes issues such as bias, fairness, privacy, and accountability.
- Key Ethical Concerns:
- Bias: AI models can perpetuate and amplify biases present in the data they are trained on, leading to unfair or discriminatory outcomes.
- Fairness: Ensuring that AI systems treat all individuals and groups fairly, regardless of their race, gender, or other characteristics.
- Privacy: Protecting individuals' privacy when AI systems collect and process personal data.
- Accountability: Establishing clear lines of accountability for the decisions made by AI systems.
- Addressing Ethical Concerns:
- Data Auditing: Identifying and mitigating biases in training data.
- Algorithmic Transparency: Making AI models more transparent and understandable.
- Ethical Guidelines: Developing ethical guidelines for the development and deployment of AI.
- Regulation: Implementing regulations to ensure that AI systems are used responsibly.
9. The Metaverse and Intelligenza Artificiale: Creating Immersive and Interactive Experiences
The metaverse, a persistent, shared virtual world, is poised to be significantly impacted by Intelligenza Artificiale. AI can enhance user experiences, personalize content, and even create entirely new virtual environments.
- Intelligenza Artificiale's Role in the Metaverse:
- Personalized Avatars: AI can create realistic and personalized avatars that reflect users' personalities and preferences.
- Intelligent Agents: AI-powered agents can act as virtual assistants, guides, or companions within the metaverse.
- Content Generation: AI can generate realistic and engaging content for the metaverse, such as landscapes, buildings, and objects.
- Social Interaction: AI can facilitate social interaction by providing real-time translation, sentiment analysis, and other tools.
10. Intelligenza Artificiale in Healthcare: Transforming Patient Care and Medical Research
Intelligenza Artificiale is revolutionizing healthcare, improving patient care, accelerating medical research, and streamlining administrative processes.
- Applications in Healthcare:
- Diagnosis and Treatment: AI can assist doctors in diagnosing diseases, recommending treatments, and monitoring patients' health.
- Drug Discovery: AI can accelerate the drug discovery process by identifying promising drug candidates and predicting their efficacy.
- Personalized Medicine: AI can personalize treatment plans based on individual patients' genetic makeup and medical history.
- Robotic Surgery: AI-powered robots can assist surgeons in performing complex procedures with greater precision and accuracy.
- Administrative Efficiency: AI can automate administrative tasks, such as scheduling appointments and processing insurance claims.
11. The Future of Work: How Intelligenza Artificiale is Reshaping Industries
Intelligenza Artificiale is transforming the future of work, automating routine tasks, augmenting human capabilities, and creating new job roles.
- Impact on Industries:
- Automation: AI is automating many routine tasks across various industries, increasing efficiency and productivity.
- Augmentation: AI is augmenting human capabilities, allowing workers to focus on more creative and strategic tasks.
- New Job Roles: AI is creating new job roles in areas such as AI development, data science, and AI ethics.
- Preparing for the Future of Work:
- Upskilling and Reskilling: Workers need to acquire new skills to adapt to the changing demands of the job market.
- Focus on Soft Skills: Soft skills such as communication, collaboration, and critical thinking will become increasingly important.
- Embrace Lifelong Learning: Workers need to embrace lifelong learning to stay relevant in a rapidly evolving technological landscape.
12. Democratization of Intelligenza Artificiale: Making AI Accessible to Everyone
The democratization of Intelligenza Artificiale refers to the trend of making AI tools and technologies more accessible to a wider range of users, regardless of their technical expertise or resources.
- Key Factors Driving Democratization:
- Cloud Computing: Cloud platforms provide affordable and scalable access to AI infrastructure and services.
- Open-Source Tools: Open-source AI frameworks and libraries make it easier for developers to build and deploy AI applications.
- No-Code/Low-Code Platforms: No-code/low-code platforms allow users to build AI applications without writing any code.
- AI Education and Training: Online courses and training programs are making AI education more accessible.
- Benefits of Democratization:
- Innovation: Democratization fosters innovation by enabling more people to experiment with and develop AI solutions.
- Economic Growth: Democratization can drive economic growth by creating new businesses and job opportunities.
- Social Impact: Democratization can help to address social challenges by empowering individuals and communities to develop AI solutions that meet their specific needs.
In conclusion, Intelligenza Artificiale is a rapidly evolving field with the potential to transform almost every aspect of our lives. From generative AI and natural language processing to computer vision and reinforcement learning, the latest advancements are pushing the boundaries of what's possible. As AI continues to develop, it's crucial to address the ethical considerations and ensure that it is used responsibly and for the benefit of all.